Polynomial basis functions
The piecewise multilinear approach can be significantly improved by using higher-order basis functions, such as the Lagrangian characteristic polynomials. The approximation properties of sparse grid interpolation techniques using polynomial basis functions have been studied extensively in [4], where error bounds depending on the smoothness of the function were derived.
From the one-dimensional case, we know that one should not use equidistant nodes for higher-order polynomial interpolation. This directly suggests using Chebyshev-based node distributions. Since an additional requirement of an efficient sparse grid algorithm is the nesting of the sets of nodes, the Chebyshev Gauss-Lobatto nodes are clearly the best choice, and are therefore also suggested in [4]. In this toolbox, this grid type (CGL) is selected by the value "Chebyshev
" for the GridType
property configurable with the spset
function.
Since version 5.0.0, an additional polynomial sparse grid is available, the Gauss-Patterson sparse grid. This grid is based on the abscissae of Gauss-Patterson integration. The Gauss-Patterson formula is a nested quadrature rule that achieves a higher degree of exactness than integration at the Chebyshev Gauss-Lobatto nodes. See [9,10] for additional details.
For a detailed description of the polynomial basis functions implemented here, please see [3, ch. 3], and the references stated therein. Since version v3.2, the toolbox uses an improved construction algorithm employing the fast discrete cosine transform, see [6].
Accuracy of polynomial interpolation
From the error bounds of the univariate case, the following general error bounds depending on the smoothness of the objective function f are derived in [4]. For f in Fdk,

![]() |
![]() |
the order of the interpolation error in the maximum norm is given by

where Aq,d(f) denotes the sparse grid interpolant of f, and N denotes the number of grid points of the sparse grids of type CGL. Note that the number of grid points N of Aq,d(f) can be computed by spdim(q-d,d)
.
Number of grid points
The number of grid points of the CGL-grid is identical to the one of the Clenshaw-Curtis (CC) grid. The number of grid points of the Gauss-Patterson grid is identical to the one of the NB grid.
The following graph illustrates the sparse grids of level 0 and level 2 of the CGL-grid in two and three dimensions.
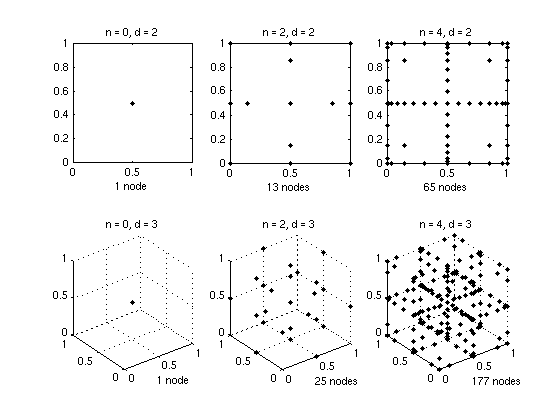
When should I use polynomial rather than linear basis functions?
There is obviously some trade-off between the accuracy gain and the computing time required to construct as well as interpolate the interpolant. Since the higher-order accuracy only becomes effective with increasing number of nodes, we recommend to use the polynomial approach only if the following two conditions are met:- The objective function to be recovered is known to be very smooth.
- High relative accuracies smaller than 10-2 are required.